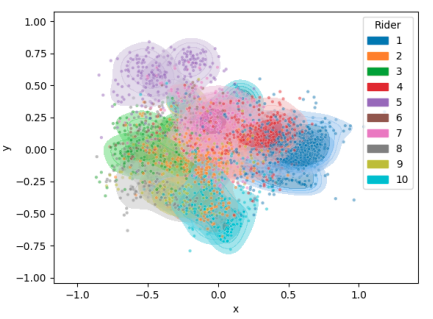
Deep Representation Learning for Personalised High Granularity Cycling Performance Prediction (2021)
NTNU
Abstract Modelling the behaviour and performance of cyclists during competition ahead of time has several useful applications to professional cycling teams. Although existing physics-based approaches perform reasonably well in this regard, they generally require several assumed parameters. Performance is also dependant on several variables which may be hard to quantify, such as a rider’s cornering ability and pacing strategy. The work presented in this thesis is a fully data-driven approach to speed pre- diction in time trials performed by elite cyclists.