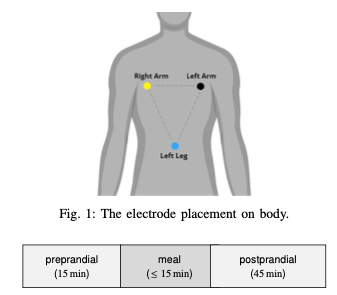
Data-Driven Classifiers for Early Meal Detection Using ECG (2023)
IEEE Sensors Letters
Abstract This letter investigates the potential of the electrocardiogram to perform early meal detection, which is critical for developing a fully-functional automatic artificial pancreas. The study was conducted in a group of healthy subjects with different ages and genders. Two classifiers were trained: one based on neural networks (NNs) and working on features extracted from the signals and one based on convolutional NNs (CNNs) and working directly on raw data. During the test phase, both classifiers correctly detected all the meals, with the CNN outperforming the NN in terms of misdetected meals and detection time (DT).