Multivariate (graph) time-series imputation
Cesare Alippi
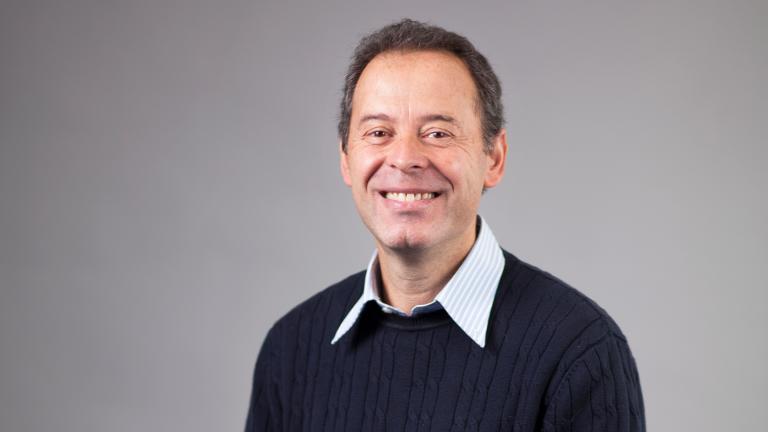
Dealing with missing values and incomplete time series is a labor-intensive and time-consuming inevitable task when handling with data coming from real-world applications, such as those related to cyberphysical systems and the Internet of Things.
Indeed, effective spatio-temporal representations allow imputation methods to reconstruct missing temporal data by exploiting information coming from sensors at different locations. However, standard methods fall short in capturing the nonlinear time and space dependencies existing within networks of interconnected sensors and do not take full advantage of the available – and often strong – relational information.
Starting from state-of-the-art imputation methods including those based on deep learning that do not explicitly model relational aspects, we gently move to graph neural networks and show how the technology can be the ultimate ground in the context of multivariate time series imputation.
About
CESARE ALIPPI received the degree in electronic engineering cum laude in 1990 and the PhD in 1995 from Politecnico di Milano, Italy. Currently, he is a Professor with the Politecnico di Milano, Milano, Italy and Università della Svizzera italiana, Lugano, Switzerland. He is a visiting professor at the Guangdong University of Technology, Guangzhou, China. He has been a visiting researcher at UCL (UK), MIT (USA), ESPCI (F), CASIA (RC), A*STAR (SIN).