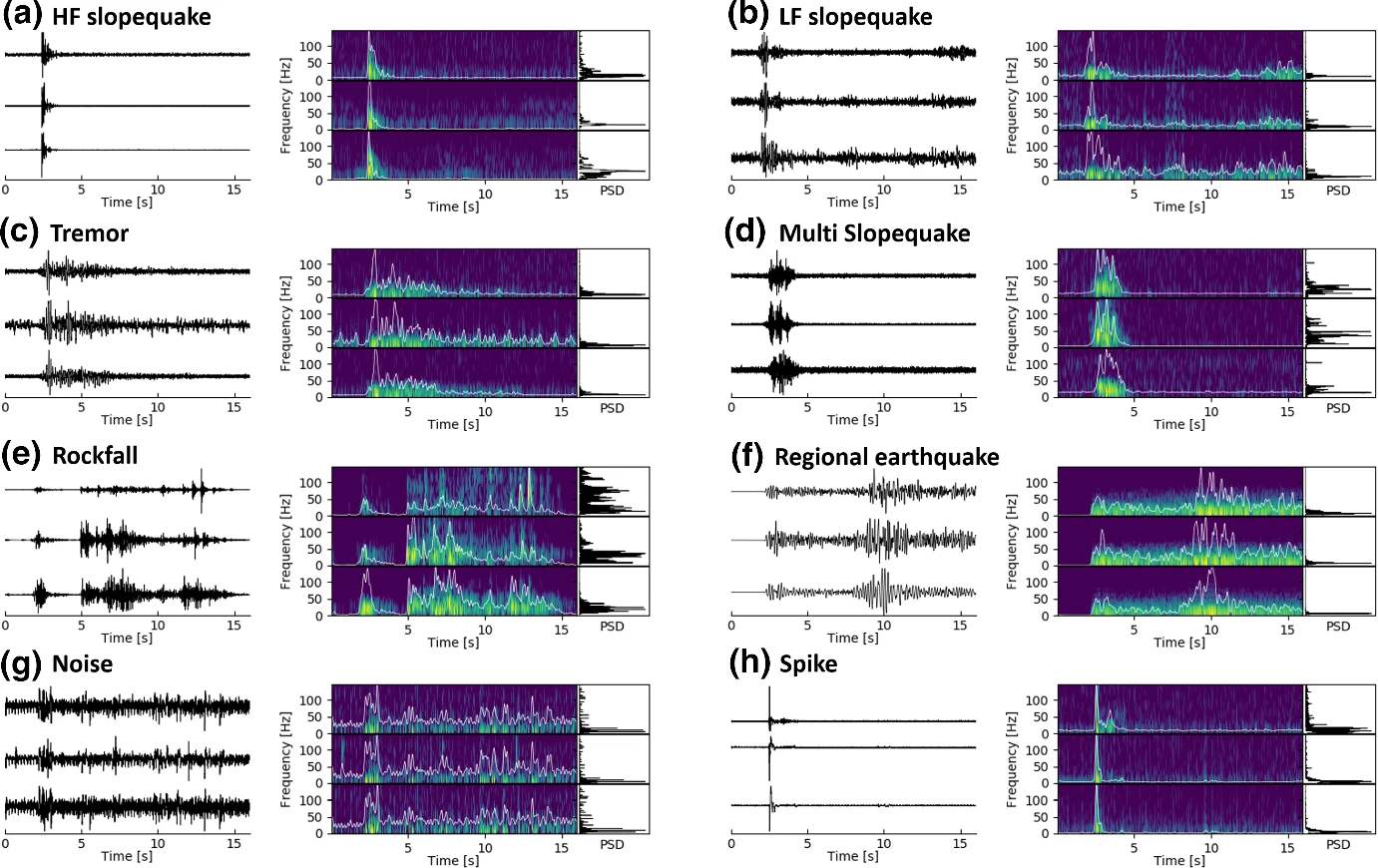
Ensemble and Self-supervised Learning for Improved Classification of Seismic Signals from the Åknes Rockslope (2022)
Mathematical Geosciences
Abstract A case study with seismic geophone data from the unstable Åknes rock slope in Norway is considered. This rock slope is monitored because there is a risk of severe flooding if the massive-size rock falls into the fjord. The geophone data is highly valuable because it provides 1000 Hz sampling rates data which are streamed to a web resource for real-time analysis. The focus here is on building a classifier for these data to distinguish different types of microseismic events which are in turn indicative of the various processes occurring on the slope.