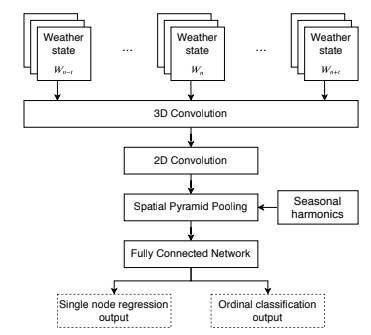
A Deep Learning-Based Method for Regional Wind Power Production Volume Prediction (2020)
NTNU
Abstract The aim of this thesis was to predict the wind power production volume of a large geographical region given the Numerical Weather Prediction data (NWP) over the region using deep learning. Accurate production volume predictions is important for power grid balancing, production planning, and price estimation. Having an accurate forecast for the upcoming wind power production volume has become more and more important in the past years due to the fast increasing number of installed wind turbines and installed total production capacity.