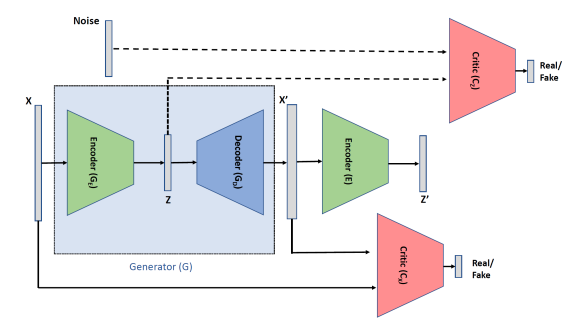
Generative Adversarial Networks for Unsupervised Anomaly Detection in Multivariate Time-Series Telecommunications Data (2021)
NTNU
Abstract In the telecommunications domain, as network infrastructure grows more complex, it is becoming increasingly important to develop systems that are able to automate efficient and accurate anomaly detection. Such systems operate on Key Performance Indicators (KPIs) collected from network base stations, and are vital to alert for possible critical operational incidents in a timely manner. These KPIs are most commonly in the form of multivariate time-series, which are challenging to perform anomaly detection on, as anomalous patterns occurs both as correlations between features, as well as temporal windows.